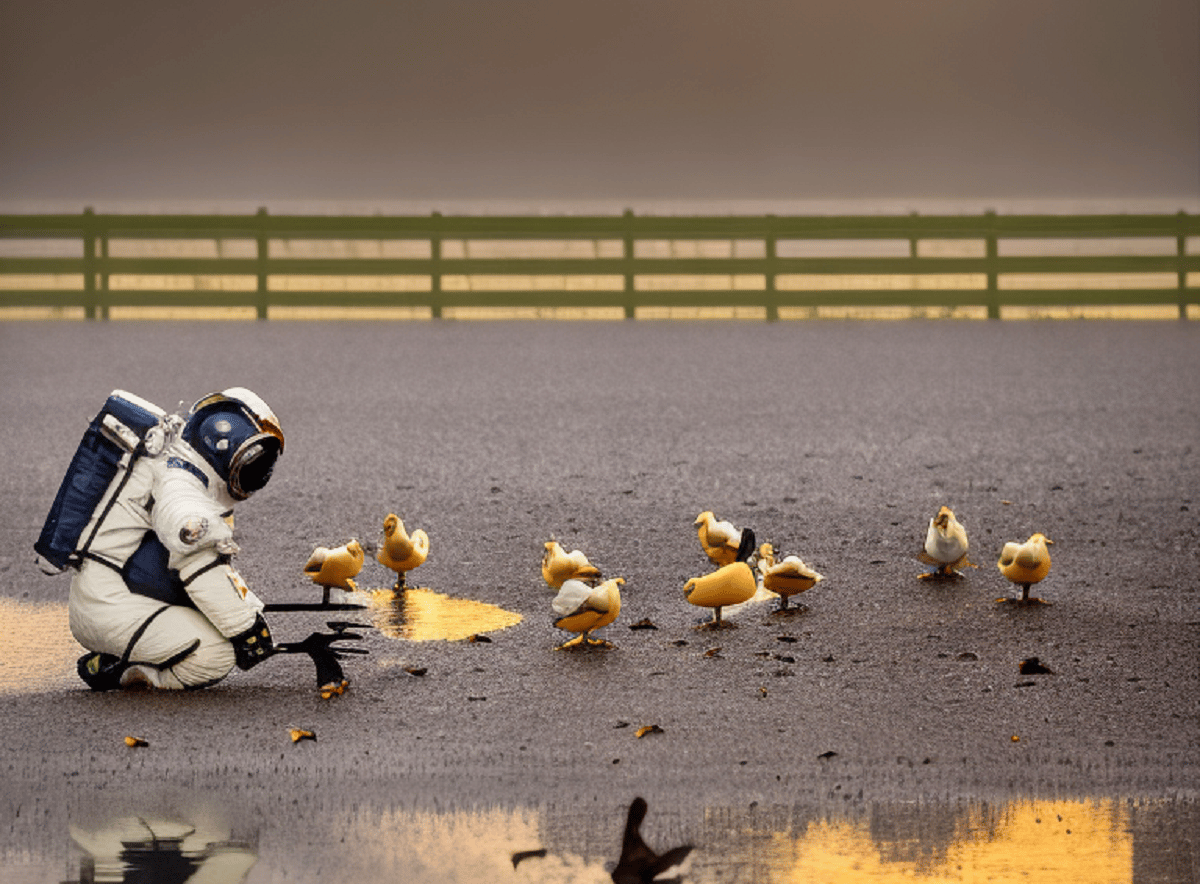
Image generated with Stable Diffusion 2.0
Recently Stability AI, unveiled via a blog post the second edition of the system automatic learning stable diffusion, which is capable of synthesizing and modifying images based on a suggested template or a natural language text description.
Stable Diffusion is a machine learning model developed by Stability AI to generate high-quality digital images from natural language descriptions. The model can be used for different tasks, such as generating text-guided image-to-image translations and image enhancement.
Unlike competing models like DALL-E, Stable Diffusion is open source1 and does not artificially limit the images it produces. Critics have raised concerns about the ethics of AI, claiming that the model can be used to create deepfakes.
The dynamic team of Robin Rombach (Stability AI) and Patrick Esser (Runway ML) from the CompVis Group at LMU Munich headed by Prof. Dr. Björn Ommer, led the original release of Stable Diffusion V1. They built on their previous lab work with latent diffusion models and gained critical support from LAION and Eleuther AI. You can read more about the original release of Stable Diffusion V1 in our previous blog post. Robin is now leading the effort with Katherine Crowson at Stability AI to create the next generation of media models with our broader team.
Stable Diffusion 2.0 offers a number of great improvements and features compared to the original V1 version.
Main news of Stable Diffusion 2.0
In this new version that is presented a new image synthesis model based on text description has been created "SD2.0-v", which supports generating images with a resolution of 768×768. The new model was trained using the LAION-5B collection of 5850 billion images with text descriptions.
The model uses the same set of parameters as the Stable Diffusion 1.5 model, but differs by the transition to the use of a fundamentally different OpenCLIP-ViT/H encoder, which made it possible to significantly improve the quality of the resulting images.
A has been prepared simplified version of SD2.0-base, trained on 256×256 images using the classical noise prediction model and supporting the generation of images with a resolution of 512×512.
In addition to this, it is also highlighted that the possibility of using supersampling technology is provided (Super Resolution) to increase the resolution of the original image without reducing quality, using spatial scaling and detail reconstruction algorithms.
Of the other changes that stand out from this new version:
- The provided image processing model (SD20-upscaler) supports 4x magnification, allowing images with a resolution of 2048×2048 to be generated.
- Stable Diffusion 2.0 also includes an Upscaler Diffusion model that improves image resolution by a factor of 4.
- The SD2.0-depth2img model is proposed, which takes into account the depth and spatial arrangement of objects. The MiDaS system is used to estimate the monocular depth.
- New text-driven interior paint model, fine-tuned on the new Stable Diffusion 2.0 text-to-image base
- The model allows you to synthesize new images using another image as a template, which may be radically different from the original, but retains the overall composition and depth. For example, you can use the pose of a person in a photo to form another character in the same pose.
- Updated model for modifying images: SD 2.0-inpainting, which allows using text hints to replace and change parts of the image.
- The models have been optimized for use on mainstream systems with a GPU.
Finally yes you are interested in knowing more about it, you should know that the code for the neural network training and imaging tools is written in Python using the PyTorch framework and released under the MIT license.
Pre-trained models are open under the Creative ML OpenRAIL-M permissive license, which allows commercial use.
Source: https://stability.ai